Algorithms and Statistical Models Vs Human Judgement
This is one of our free-to-access content pieces. To gain access to all Ideas for Leaders content please Log In Here or if you are not already a Subscriber then Subscribe Here.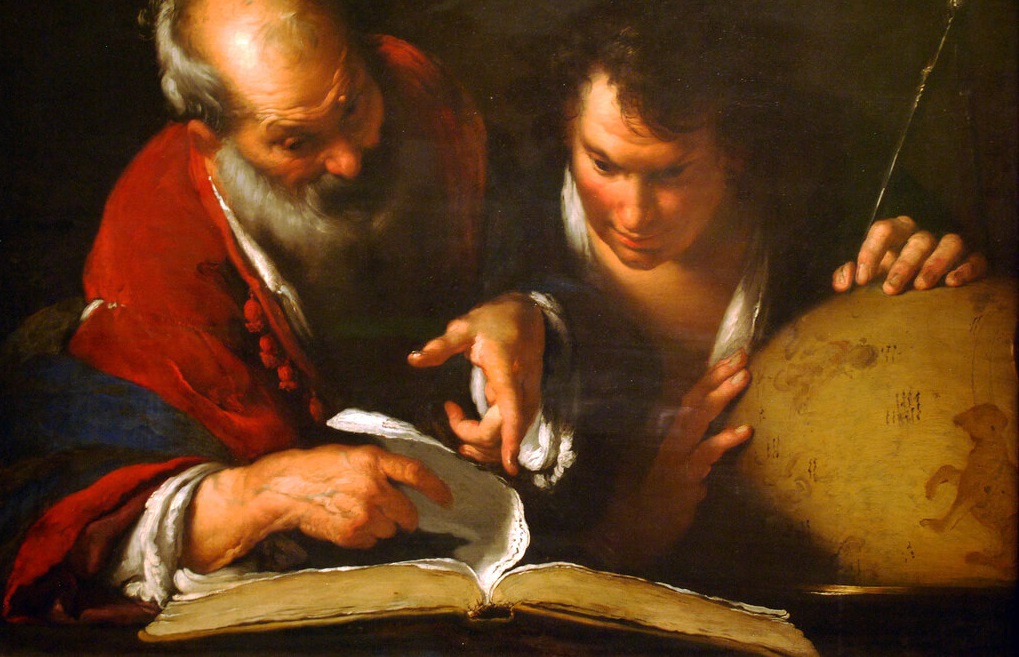
For businesses frustrated by algorithm aversion — the tendency of people to reject forecasts based on algorithms and statistical models in favour of less dependable human judgement — there is hope: a new study shows that people will choose to use algorithms if they can modify them, even slightly.
Decades of research have shown that algorithms (defined here as any evidence-based forecasting formula, including statistical models) are more accurate than forecasts made by humans. However, research has also shown that most people have greater confidence in their ability to make forecasts rather than leaving it to mathematical formulae — an attitude known as ‘algorithm aversion.’
Algorithm aversion is more nuanced than simply refusing to believe in the power of mechanical processes over human judgement. People reject algorithms because they judge them more harshly than they judge humans. In other words, although forecasts based on algorithms are significantly more accurate than human forecasts, they are not perfect. Once this ‘imperfection’ is known, people immediately prefer to depend on human judgement — even though the performance of even ‘imperfect’ algorithms is better.
At first glance, this somewhat illogical aversion to imperfect algorithms can make business leaders pessimistic about their chances to get employees to use them. However, if ‘imperfection’ is the stumbling block, there is a potential resolution to the impasse: to allow people to make some adjustments to the figures received from the algorithms if they believe the figures are off.
Building on this idea, a team of researchers — who had identified algorithm aversion in prior research — conducted a series of studies to see whether the opportunity to ‘correct’ the imperfections of algorithms would lead to greater use.
All of the studies used the same task: predicting students’ scores on a standardized test based on a set of factors, from socio-economic status and expected highest degree to how many friends are not going to college. Participants had access to an actual statistical model that predicts student scores based on these criteria. The researchers then created different situations to answer different questions, such as whether allowing people to make limited or unlimited adjustments to a statistical model’s results would spur them to use the model more.
For example, all participants in the first experiment had to choose between using the model or using their own judgement exclusively. For some of the participants, choosing the model meant accepting all of the model’s figures without any changes. Other participants who decided to use the model were allowed to change the figures up to 10%. Still other participants who used the model could adjust the figures as much as they wanted… but for only 10 of the 20 predictions they were given.
Based on the data collected from this series of studies, the researchers confirm that allowing employees to adjust the results of statistical models and other algorithms can indeed overcome algorithm aversion. One surprising result of the study is that the size of the adjustment is irrelevant. In other words, it does not seem to matter how much people can adjust the results of a statistical model, as long as they can make some changes. In one study, for example, whether participants were allowed to change the figures by 10%, 5% or 2% made almost no difference: as long as it was not 0%, the participants were willing to use the model.
The bottom line is that people don’t want to be at the complete mercy of an algorithm. Even the most constrained freedom of adjustment breaks the ice, and makes them not only more likely to use a model, but also makes them:
This study has clear implications for managers wanting their employees and customers to rely more on algorithms. The first lesson: do not make it an all-or-nothing decision. In other words, telling employees they must choose one or the other option exclusively is counter-productive. Once they have some feedback about the algorithm and learn that is not perfect, they will probably not be willing to commit to the algorithm’s results.
However, they will be more likely to choose to use the algorithm if you give them a chance to modify its forecasts.
To ensure the best results, let people adjust forecasts only to a limited degree, if possible. Limited adjustments will ensure that the bulk of the algorithm’s (more accurate) results stay, while still giving employees some control over the process.
If for some reason, constrained adjustments are not possible, even allowing them unconstrained flexibility on the results — that is, they can change the figures at will — will lead in the long run to better forecasting performance.
Ideas for Leaders is a free-to-access site. If you enjoy our content and find it valuable, please consider subscribing to our Developing Leaders Quarterly publication, this presents academic, business and consultant perspectives on leadership issues either as a digital subscription, or better still in a beautifully produced, small volume delivered to your desk four times a year.
For the less than the price of a coffee a week you can read over 650 summaries of research that cost universities over $1 billion to produce.
Use our Ideas to:
Speak to us on how else you can leverage this content to benefit your organization. info@ideasforleaders.com