Exploration Algorithms Increase Diversity of New Hires
This is one of our free-to-access content pieces. To gain access to all Ideas for Leaders content please Log In Here or if you are not already a Subscriber then Subscribe Here.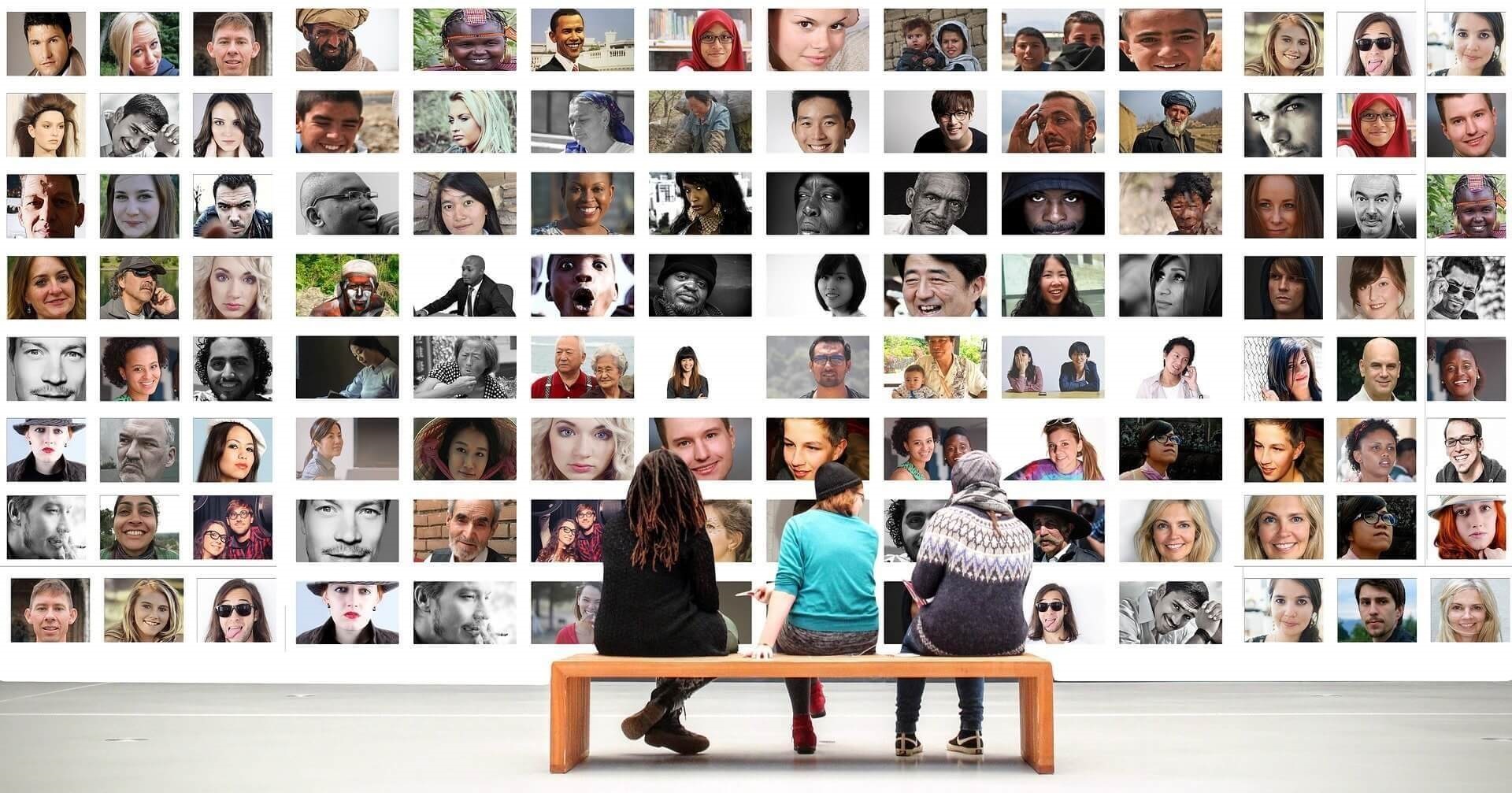
The use of hiring algorithms willing to ‘explore’ job candidates with profiles that differ from past successful candidates increases the diversity of a firm’s workforce, a new study shows.
More and more firms with ongoing recruitment of professionals use computer algorithms to screen job applicants. The screening process is based on past history: the algorithm compares a candidate’s profile with the profiles of past successful candidates—success in terms of being selected for an interview and success in terms of accepting an eventual job offer.
The flaw in this past history or ‘exploitation’ approach is that as the algorithm continues to select candidates that match the profile of past candidates, the firm never interviews or hires different types of people. This results in underrepresented profiles—based on demographics, education, or work history, for example—in the candidates the firm interviews or hires. For example, if few women were interviewed or hired in the past, few women will be interviewed or hired in the future. Although there are two types of exploitation algorithms—static supervised learning (static SL) based on data that never changes, and updating supervised learning (updating SL) based on data periodically updated—the vicious circle is unbroken: the choices of the present generally mirror the choices of the past.
MIT researchers developed an algorithm that overcomes this flaw by approaching the recruitment of new hires as a contextual bandit problem. Taking its name from an analogy of slot machines (so-called ‘one-armed bandits’), a contextual bandit problem refers to a problem that involves uncertainty and multiple options. Research has shown that optimal results occur when exploitation, making choices based on what has worked in the past, is balanced with exploration—choosing alternatives that you know little about in order to learn more about those alternatives. For example, gamblers win more when they play the slot machines they know will be winners but also take chances with slot machines they are unsure of.
In terms of recruitment, a contextual bandit approach to hiring translates as selecting candidates that fit the profile of past interviewees and hires (exploitation), but also deliberately selecting candidates that don’t fit the profile of past successful candidates in order to learn more about their potential for success (exploration).
To test the effectiveness of their contextual bandit algorithm, the MIT researchers modelled their algorithm against traditional exploitation algorithms and human recruiters using recruitment data based on 40 months of hiring at a professional services firm. This dataset included nearly 90,000 job applicants, of whom less than 5,000 were chosen for an interview, and less than 500 were eventually hired by the firm.
Most applicants in the dataset were male (68 percent) and either Asian (58 percent) or white (29 percent). Only 13 percent of applicants and 5 percent of new hires were Black or Hispanic. While representing just 32 percent of the applicants, 34 percent of new hires in the dataset were women.
The modelling yielded the following results:
The results of the hiring rates show the updating SL outperforming the contextual bandit exploration algorithm. However, the hiring rates in the algorithm models come with a constraint: there is no data available on the hiring rates of the candidates that the algorithms would have selected for interviews since they were never interviewed by the human recruiters (and thus never had the opportunity to be hired). When the researchers conducted a simulation that eliminated this constraint, they found that the exploration model learned more quickly than the updating SL algorithm about the increased hiring rates of blacks and Hispanics, which led in turn to a greater number of black and Hispanics being selected for interviews.
The result is significant: a black applicant would have a 2 percent chance of being interviewed if the application was processed by an updating SL algorithm versus a 10 percent chance of being interviewed if the contextual bandit algorithm was used.
This research has implications related to recruitment efficiency and effectiveness, notably in the hiring of a more diverse workforce.
Based on the results of this study, firms receiving a flood of job applications can benefit from the processing speed of algorithms without sacrificing the quality of the candidates interviewed and eventually hired. The algorithms in this study selected candidates that were more likely to receive and accept an offer than the candidates selected by the firm’s human recruiters.
Many professional services firms that struggle with increasing the diversity of their workforce will find that algorithms can improve results in this area as well. However, while all algorithms outperform human recruiters in increasing diversity, this study indicates that algorithms that combine an exploitation and exploration approach as opposed to the exploitation approach only would yield the best results.
Ideas for Leaders is a free-to-access site. If you enjoy our content and find it valuable, please consider subscribing to our Developing Leaders Quarterly publication, this presents academic, business and consultant perspectives on leadership issues in a beautifully produced, small volume delivered to your desk four times a year.
For the less than the price of a coffee a week you can read over 650 summaries of research that cost universities over $1 billion to produce.
Use our Ideas to:
Speak to us on how else you can leverage this content to benefit your organization. info@ideasforleaders.com